When we talk about “omics,” we are referring to all the things that happen in a certain layer of human biology.
There are a lot of omics out there, from genomics, to transcriptomics, to proteomics and so on – the list is extensive. And when we talk about these omics in the context of research, we are looking at measuring a vast number of things.
What we are facing is layer upon layer of data – tens of thousands to millions of data points per subject. The challenge at hand is: How do we make sense of all this data that traditional math, statistics and informatics are not powerful enough to cope with? The answer is data science and artificial intelligence.
Connecting omics to AI
My background is a mixture of bioinformatics and artificial intelligence. I bridged the two camps more than a decade ago because I was passionate about understanding how these fields could marry together and produce significant value for humanity. Now, one of the challenges for the biopharmaceutical industry nowadays is to understand how omics work with AI. Let’s look at genomics (the study of all the genes in the genome) to explain why it is of such importance
In genomics, we are interested in the variations across the entire genome, which can reach as many as eight million variations of humans. Translated into data, that’s eight million data points. Then imagine we are looking at the entire population of Australia, where I live and work. We are now talking about 8 million data points across 25 million people. That is probably more data than humanity has ever witnessed in history – and it is just for the genome layer.
Our purpose as data science researchers is to connect data points like this together from all the omics and non-omics, to discover how diseases are caused and how we could prevent or cure them. However, the data volume has become so great that classical mathematical modeling is no longer powerful or efficient enough. This is where AI comes in.
" With AI we can improve our understanding of diseases and at a much higher resolution than ever. "
By Monther Alhamdoosh, CSL Director, Head of Bioinformatics and AI at Global Research Data Science
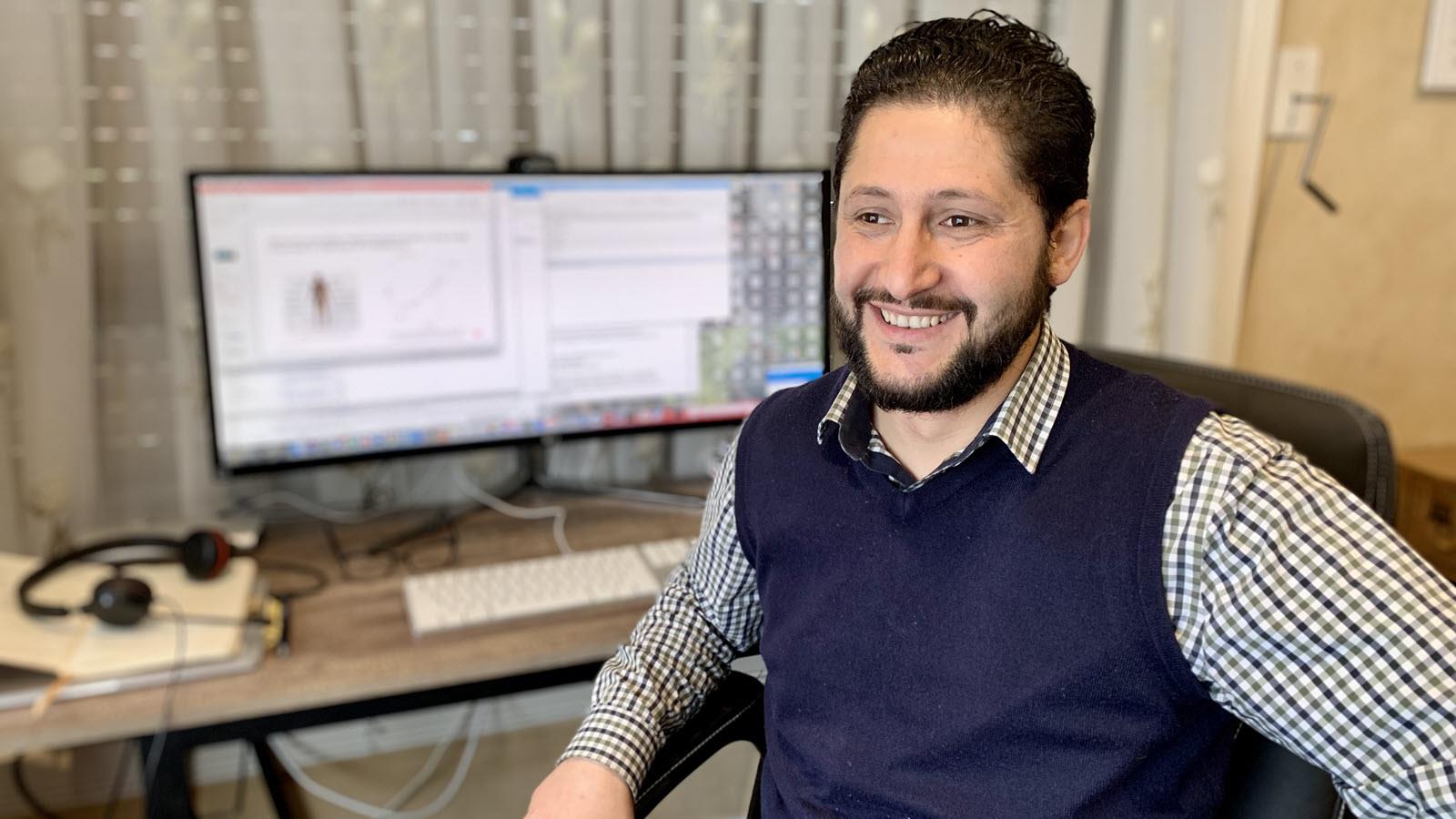
How we are utilizing AI in the biopharmaceutical industry
Putting all this information together requires machines. Humans cannot do it. But one of the key problems we address in AI is knowledge representation: how to enable the machine to understand all this mixed data combined with what is already established in academic literature.
Therefore, our focus is on building relationships between these data points and what is already out there, to help machines learn from this data and make predictions or hypotheses. After a hypothesis is generated, we can take it into the lab for validation and it is much faster and easier from there. So, there is a lot of potential for data science and AI to accelerate drug discovery.
By integrating AI and omics, we will also at some point in the future be able to understand complex diseases that we do not presently, such as idiopathic pulmonary fibrosis, rheumatoid arthritis and cardiovascular diseases. We need to collect and analyze enormous amounts of data for this, and the AI field is now mature enough to help us do that.
How AI is changing the way we work to empower reverse translational medicine
Technologies are evolving fast. We can generate more data than ever, and we are collaborating more than ever, which is pushing the biopharmaceutical industry to change its approach to developing therapeutics. With AI we can improve our understanding of diseases and at a much higher resolution than ever. We can ascertain with more confidence how or why a drug is likely to treat certain diseases effectively and move it into clinic quickly. We can get there faster, evaluate faster and relaunch faster when needed.
This allows reverse translation, when we take insights from the bedside back to the bench to understand why certain patients did not respond to therapy and what can/should be done to improve our chances of success. The goal is to deliver more personalized treatments to our patients rather than one-size-fit-all treatments. Data science and artificial intelligence will give us the flexibility and speed we need to get there.